We don't really understand AI
Despite the breathtaking progress to date, we don't fully understand how generative AI works.
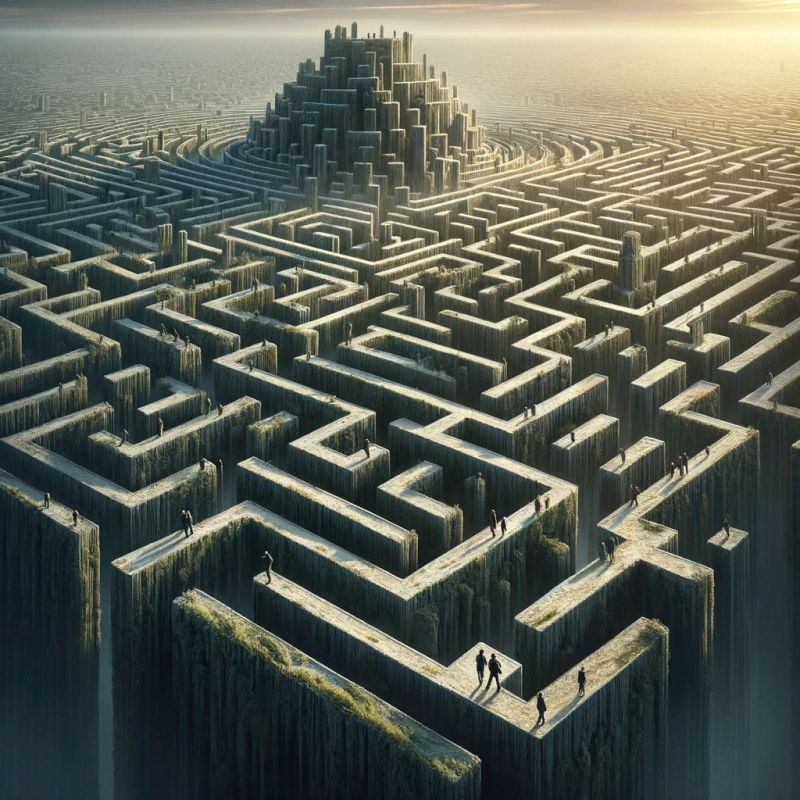
Despite the breathtaking progress to date, we don't fully understand how generative AI works.
I made this remark at a closed-door panel discussion I moderated last week, alongside representatives from Microsoft and Nvidia.
Nobody disagreed.
๐ ๐ง๐ต๐ฒ ๐๐ฟ๐๐๐ต ๐ฎ๐ฏ๐ผ๐๐ ๐๐
While hardly news to those dabbling in AI, many will probably be surprised by this revelation.
Over the weekend, I came across an article in MIT Technology Review that offered the clearest explanation of the mystery of LLMs yet.
Here's my summary.
๐ ๐ญ/ ๐๐ ๐บ๐๐๐๐ฒ๐ฟ๐
By accident, two researchers at OpenAI discovered a phenomenon where models would seemingly fail to learn a task, then get it all of a sudden.
But this wasn't how deep learning was supposed to work; it was but one of several odd behaviours that had AI researchers scratching their heads.
โObviously, weโre not completely ignorant. But our theoretical analysis is so far off what these models can do... I think this is very mysterious,โ said a computer scientist.
An AI researcher was quoted saying: "My assumption was that scientists know what theyโre doing. Like, theyโd get the theories and then theyโd build the models. That wasnโt the case at all."
โIt was like, here is how you train these models and then hereโs the result. But it wasnโt clear why this process leads to models that are capable of doing these amazing things.โ
๐ ๐ฎ/ ๐ง๐ผ๐ผ ๐ฐ๐ผ๐บ๐ฝ๐น๐ฒ๐ ๐๐ผ ๐บ๐ผ๐ฑ๐ฒ๐น
The rapid advances over the last decade came more from trial and error than from understanding, as researchers copied what worked and added their own innovations.
Said another computer scientist: โWe have a lot of experimental results that we donโt completely understand, and often when you do an experiment it surprises you.โ
For now, the biggest models are now so complex that researchers are experimenting on smaller varieties of statistical models as a proxy.
And each time that the basics get nailed down, breakthroughs would disprove it: "And then people made models that could speak 100 languages and it was like, okay, we understand nothing at all.โ
โIt turned out we werenโt even scratching the surface.โ
โ๏ธ ๐ฏ/ ๐ฆ๐ฎ๐ณ๐ฒ๐๐ ๐ถ๐ ๐๐ต๐ฒ ๐ถ๐๐๐๐ฒ
Ultimately, the greatest issue is safety. Without a complete understanding of the theory behind the science, how can we predict the capabilities that may emerge?
Put another way, one won't know what GPT-5 or GPT-6 is capable of until it is trained and tested. But what if it goes rogue? How would we detect it and stop it in time?
For now, work continues apace to explain the capabilities of our existing models.
And yes, a "superalignment" team at OpenAI was also set up to figure out how we might stop a hypothetical superintelligence.
๐๐บ๐ฎ๐ด๐ฒ ๐๐ฟ๐ฒ๐ฑ๐ถ๐: DALL-E 3