The future of AI is murkier than before
When the only certainty is that AI will be everywhere.
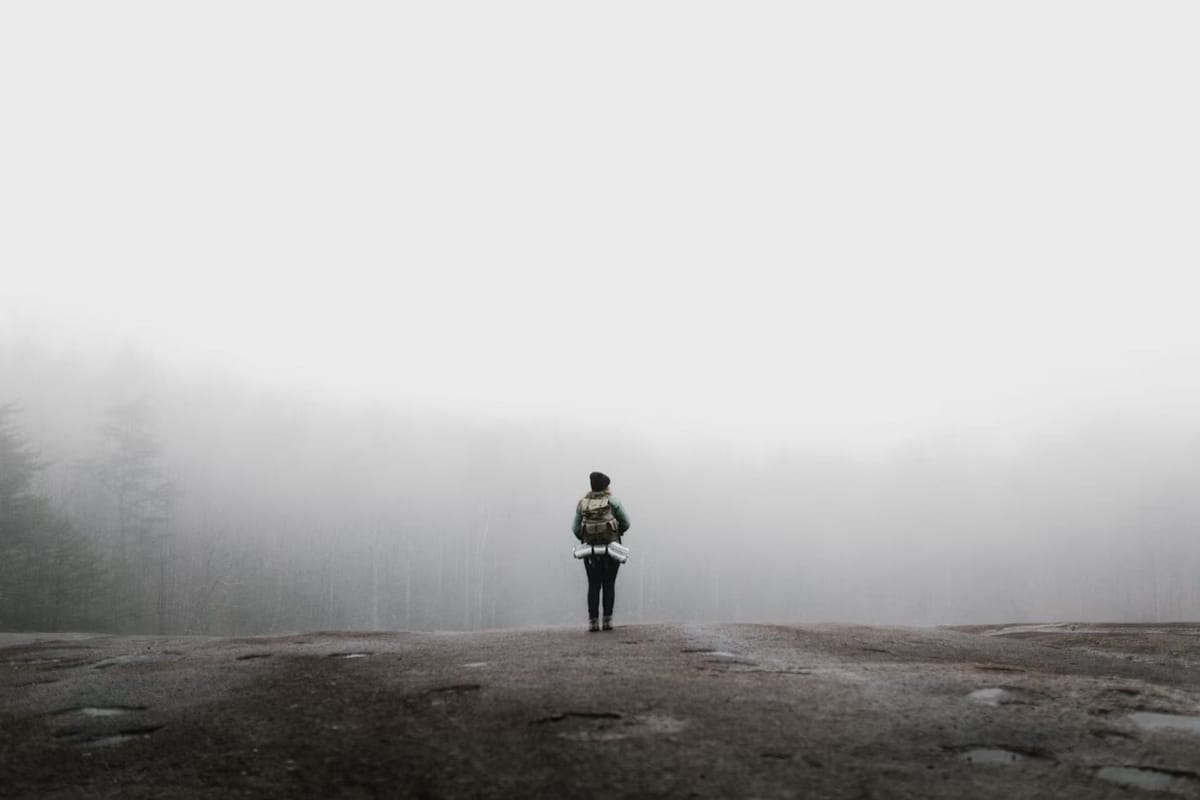
Will AI put us out of jobs? How can we adapt to the changes it will bring? These are some of the perennial topics we see splashed across headlines as the world grapples with the profound implications of generative AI.
In the meantime, AI continues to advance at an astounding pace, with larger, smarter, and more versatile models, sometimes with competing models released a week or mere days apart. And tech firms such as OpenAI, Meta, and even late-comer xAI are gearing up for the next leap ahead with 100,000 GPU clusters.
Yet as we approach the two-year mark since the public availability of ChatGPT, the impact of AI appears as unclear as it was the day after its release. For the man on the street, the result is an increased sense of uncertainty about the road ahead.
Jobs already impacted
Two weeks ago, I wrote that job losses have already begun, based on data-centric studies that found certain freelance jobs requiring basic writing, coding, or translation are drying up.
I cited a Wall Street Journal report with anecdotes from two freelancers who saw long-time writing clients leave and of fees offered for new gigs halved. Both are strong indicators of generative AI disrupting work at an accelerating rate.
Freelance workers matter because they make up a significant proportion of the workforce, including in Singapore. And while full-time roles are generally more resilient against displacement by AI, the value of these roles will inevitably weaken over time. In a way, the job prospects of freelancers represent the canary in the coal mine for the broader economy.
So, are businesses turning to generative AI and firing workers en masse? This is where the picture gets decidedly murky.
Harder than expected
In its latest study to assess generative AI readiness and implementation in businesses, management consulting firm Bain & Company observed that businesses are only just learning how difficult it is to implement generative AI in their organisations.
They cited responses from tech firms – which are generally first movers in adopting new technologies – which indicated a decline in confidence in the readiness of their data, resources, and policies for generative AI compared to an earlier survey. In comparison, industries in other industries reported the same level of readiness.
As organisations rush to implement AI in everything, it is also telling that not all pilots succeed. For now, some success is reported in use cases around sales and sales operations, software code development, marketing, customer service, and customer onboarding. Conversely, use cases in legal, operations, and HR appear less successful.
Not every problem can be solved with AI. At a recent private roundtable discussion I facilitated, one senior tech leader expressed discomfort at the pressure exerted by business leaders to quickly harness generative AI. This leader stressed the importance of finding the right use case and a successful proof of concept (POC).
To be clear, companies are investing heavily in generative AI, and are spending as much as US$5 million to US$50 million annually, according to Bain. Based on self-reported data, nine out of 10 (87%) are already developing, piloting, or deploying generative AI in some capacity right now.
Why AI projects fail
As we rush headlong to deploy AI, one possible catalyst for failure might be our tendency to overestimate what it can do. But why does this happen? According to MIT robotics pioneer Rodney Brooks, this is due to our tendency to extrapolate what AI can do using our understanding of humans.
When we see an AI system perform a task, we subconsciously generalise it using similar experiences. We then estimate the overall competence of the AI system. This is similar to how we sometimes gauge a person's competency based on how they dress and speak, or how well they did for a given company project. Ironically, it is our human bias that leads us astray.
Our overexuberance is likely another issue. Not every problem is a nail, and generative AI isn't the hammer to solve everything. On this point, Rodney referred to well-meaning advice to leverage large-language models (LLMs) for the warehouse robots his robotics startup makes, noting that language will likely "slow things down" compared to the precise, sophisticated algorithms that are currently used.
AI will be everywhere
Not everything is murky though. If there is one thing that is crystal clear, it would be that generative AI is here to stay.
Around the world, technology firms continue to pour vast amounts of resources and spend untold billions on AI. Many are offering their models for commercial access, while some, such as Meta, have made certain models available for open-source access. Yet others have released tiny models that can run off smartphones.
Even if AI training were to stop today, or we discover in the months ahead that we have already reached the pinnacle of what is possible with deep learning, the many models that we have already trained are more than enough to revolutionise industries and reshape the future.
There is no stuffing AI back into the box.
The only question is how we plan to harness its capabilities navigate the societal challenges that would doubtlessly emerge. And finally, how we plan to upskill individually to stay relevant in the days ahead.